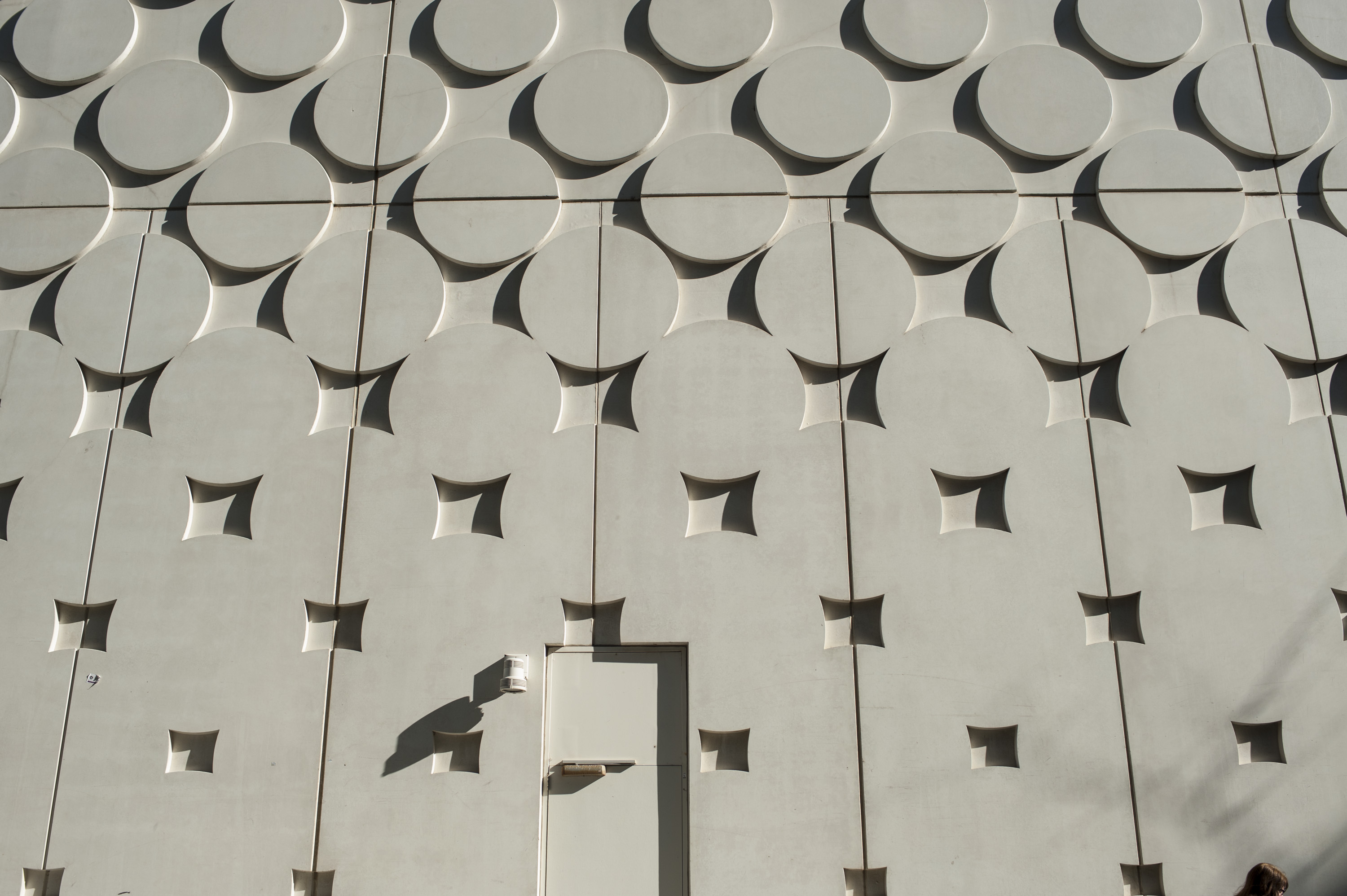
Bayesian Statistics
36 contact hours or online equivalent plus private study
One Study period
Overview
The unit introduces the fundamentals of Bayesian statistical modelling. Students will learn the importance of subjective beliefs in Bayesian statistics. Important concepts such as prior distributions, likelihood functions, and posterior distributions will be discussed at length. Numerical estimation techniques, such as Metropolis-Hastings and Gibbs sampling, will be introduced. Empirical applications of Bayesian analysis will be performed in an R software environment.
Requisites
Teaching Periods
Location
Start and end dates
Last self-enrolment date
Census date
Last withdraw without fail date
Results released date
Learning outcomes
Students who successfully complete this unit will be able to:
- Differentiate important distributions commonly used in Bayesian Statistics
- Defend the importance of concepts such as Prior Distributions and Posterior Distributions in Bayesian Statistical Modeling
- Describe the importance of Markov Chain Monte Carlo simulation in Bayesian Analysis
- Develop programming capabilities to perform Bayesian analysis
- Evaluate empirical applications of Bayesian analysis in an appropriate software environment
- Articulate the differences between Bayesian estimation and maximum likelihood estimation
- Argue the merits of Bayesian methodology
Teaching methods
Hawthorn Online
Type | Hours per week | Number of weeks | Total (number of hours) |
---|---|---|---|
Online Directed Online Learning and Independent Learning | 12.50 | 12 weeks | 150 |
TOTAL | 150 |
Assessment
Type | Task | Weighting | ULO's |
---|---|---|---|
Assignment 1 | Individual | 20% | 4,5,7 |
Assignment 2 | Individual | 20% | 4,5,7 |
Examination | Individual | 50% | 2,3,6,7 |
Online Quiz | Individual | 10% | 1,2 |
Content
- Bayes theorem and the concept of Bayesian statistics
- Distributions often used in Bayesian statistics
- Prior distributions, likelihood functions, and posterior distributions
- Bayesian estimation and model fitting using appropriate software
- Differences between Bayesian estimation and maximum likelihood estimation
- Choice of prior distribution
- Empirical Bayes estimation
- Bayesian hierarchical models
- Empirical applications of Bayesian analysis using appropriate software
Study resources
Reading materials
A list of reading materials and/or required textbooks will be available in the Unit Outline on Canvas.